Study finds microscopic imaging devices may gather data on material structure
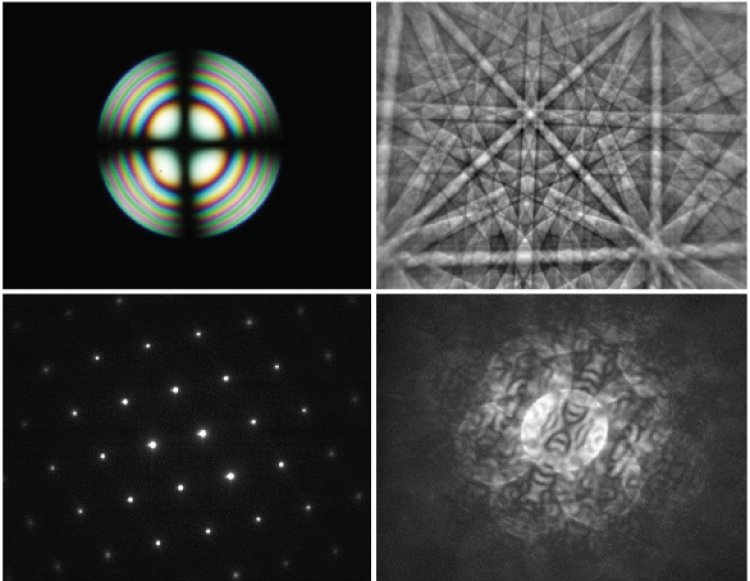
Washington DC, US: Researchers have created a technique that uses machine learning with a human in the loop to automatically recognise and measure a material's microstructure from microscopic photographs.
From the nanoscale to the mesoscale, microscopic imaging devices may gather data on material structure at a variety of scales.
Quantitative analysis of microstructure is the method of extracting structural information from microscopic images. The complexity and diversity of microstructure, however, have placed some restrictions on how well humans and AI have been able to do this on their own.
The research team successfully combined human and AI capabilities to establish an integrated framework for quantitative microstructure analysis. With the help of a single microstructure image and the relevant scribbling annotation of subject-matter experts, this method enables AI to carry out microstructure segmentation.
Additionally, the AI actively solicits professional inputs from scribes in order to enhance the model's functionality and dependability. Through extensive testing, the study team established that the framework of human-AI collaboration is pervasive and can be used in a variety of materials, microstructures, and microscopic imaging systems.
Prior to this study, massive amounts of dense annotation were required for previous research; however, by replacing dense annotation with scribbling annotation, which can be easily written with a pen or mouse, this study dramatically reduced annotation costs.
The Automated Microstructure Quantitative Analysis System (TIM) at KIMS will use this technology. This will make it easy for general researchers to use.
Dr. Juwon Na, a senior researcher at KIMS, said, “This study is the result of improving the existing subjective and time-consuming quantitative analysis of microstructure into an objective and automated process.”
Professor Seungchul Lee of POSTECH, added, “Our framework that interacts with experts is expected to be widely used as a core analysis technology in industry and research, and through this, we expect to dramatically reduce the cost and time of new materials research and development and further significantly improve reliability.”